S4S - S.W.E.A.T. for Science

Research project at a glance
Departments and Instituts
Period
23.10.2014 to 23.02.2018
Project manager at H-BRS
Project Description
The question of how to produce effective and appropriate physical training plans is of great importance in many areas. For both professional and amateur athletes, getting the most out of every training session and progressing toward a long term fitness goal is of obvious interest. Even more important is the building of physical fitness for the less able-bodied. For cardiovascular patients and those in need of physical rehabilitation, the effectiveness of physical training is a quality of life issue of critical importance. Regardless of the application or goal, it is important to avoid injury or exhaustion due to training, and at the same time achieve the maximum long term performance improvement.
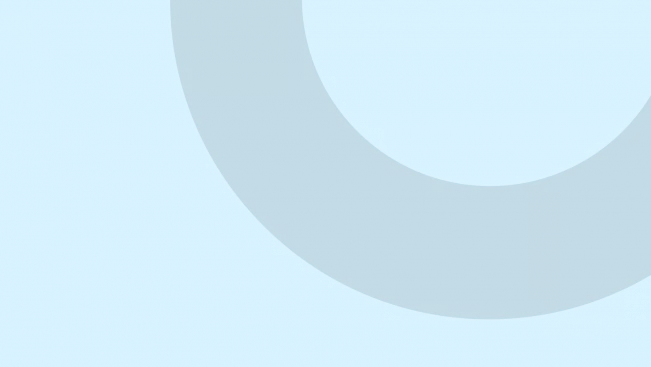
The Specific Workout, Efficient Assisted Training (S.W.E.A.T. for Science) project focuses on the modeling of training workout plans and the prediction of individual long-term performance under these plans. The aim is to automatically produce workout plans which deliver the largest long-term increase in performance, while avoiding exhaustion or unnecessary strain. These workout plans, combined with the intelligent control of electrically-assisted bicycles, facilitate appropriate training regardless of the length or ruggedness of the route, headwinds, or other factors. Based on individually tailored performance predictions and workout plans, the electric motor can provide additional assistance to prevent excessive stress, or lower the assistance to ensure adequate training.
The S.W.E.A.T. for Science project specifically addresses the improvement of endurance training in running and cycling. Existing models from the sports science literature are examined and used as a starting point, and we apply own expertise in machine learning and signal processing to modify them and to develop models of our own. As we learn more about the physiological effects of training, we can integrate this knowledge into an adaptive e-bike controller to allow professional athletes, recovering patients, and everyone in between to get the most out of their training. Additionally, motivation aspects are relevant to develop a customized interface which can meet the individual needs of cyclists and adjusts itself adaptively to the user and support reaching their personal activity goals while avoiding overloading.
Results
Theses and Projects (work in progress)
- Kevin Bach
- Lukas Birr
- Felix Hampe
- Bastian Lang
- Anniruddha Pal
- Christoph Schmid
- David Uhlig
Theses and Projects (finished)
- Kevin Kapitza: Modelling effects of physical training on human performance: a comparison of Fitness-Fatigue-Models and neural network approaches, Bachelorthesis, 2017
- David Schäfer: Trainingsportal zur Evaluation von Trainingsmodellen, Masterthesis, 2015
- Melanie Ludwig: Computational Prediction of Training on Physical Performance: Model Analysis and Comparison, Masterthesis 2015
- David Schäfer: Optimierung von Trainingsplänen auf Basis von Langzeitmodellen, Masterproject, 2015
- Melanie Ludwig: Vergleichende Evaluierung von Kurz- und Langzeit-Trainingsmodellen, Masterproject, 2014
Awards
-
Best student paper award
Melanie Ludwig, Ashok Meenakshi Sundaram, Matthias Füller, Alexander Asteroth, Erwin Prassler: On modeling the cardiovascular system and predicting the human heart rate under strain. Proceedings of the 1st International Conference on Information and Communication Technologies for Ageing Well and e-Health (ICT4AgingWell), 2015
Publications
- Melanie Ludwig, Harald Grohganz, and Alexander Asteroth. A Convolution Model for Heart Rate Prediction in Physical Exercise. In Proceedings of the 4th International Congress on Sport Sciences Research and Technology Support (icSPORTS), pages 157-164, 2016.
- Melanie Ludwig, David Schaefer, and Alexander Asteroth. Training Simulation with Nothing but Training Data - Simulating Performance based on Training Data Without the Help of Performance Diagnostics in a Laboratory. In Proceedings of the 4th International Congress on Sport Sciences Research and Technology Support (icSPORTS), pages 75-82, 2016
- Melanie Ludwig, Alexander Asteroth. Predicting Performance from Outdoor Cycling Training with the Fitness-Fatigue Model. In A. Artiga Gonzalez, R. Bertschinger, T. Dahmen, M. Gratkowski, S. Wolf, and D. Saupe, editors, Proceedings dvs-Workshop Modelling in Endurance Sports, University of Konstanz, Sept. 9–11, 2016, pages 3–6. KOPS – The Institutional Repository of the University of Konstanz, 2016.
- Matthias Füller, Ashok Meenakshi Sundaram, Melanie Ludwig, Alexander Asteroth and Erwin Prassler. Modeling and Predicting the Human Heart Rate During Running Exercise, in Information and Communication Technologies for Ageing Well and e-Health, pages 106-125, Springer, 2015
- David Schaefer, Alexander Asteroth, and Melanie Ludwig. Training plan evolution based on training models. In International Symposium on Innovations in Intelligent Systems and Applications, 2015.
- Melanie Ludwig, Ashok Meenakshi Sundaram, Matthias Füller, Alexander Asteroth, Erwin Prassler: On modeling the cardiovascular system and predicting the human heart rate under strain. Proceedings of the 1st International Conference on Information and Communication Technologies for Ageing Well and e-Health (ICT4AgingWell), 2015